Estimating aboveground carbon stocks of urban trees by synergizing ICESat-2 LiDAR with GF-2 data |
| |
Affiliation: | 1. State Key Laboratory of Urban and Regional Ecology, Research Center for Eco-Environmental Sciences, Chinese Academy of Sciences, Beijing 100085, China;2. School of Life Sciences, Division of Life Sciences and Medicine, University of Science and Technology of China, Hefei 230027, China;3. University of Chinese Academy of Sciences, Beijing 100049, China;4. Beijing-Tianjin-Hebei Urban Megaregion National Observation and Research Station for Eco-Environmental Change, Research Center for Eco-Environmental Sciences, Chinese Academy of Sciences, Beijing 100085, China.;5. Rural Energy and Environment Agency, Ministry of Agriculture and Rural Affairs, Beijing, 100125, China;6. State Key Laboratory of Severe Weather, Chinese Academy of Meteorological Sciences, Beijing, China;1. University of Maryland, Department of Geographical Sciences, College Park, MD 20742, USA;2. Sigma Space Corp., Lanham, MD, 20706 USA;3. Code 618, Biospheric Sciences Branch, NASA/Goddard Space Flight Center, Greenbelt MD 20771, USA;4. Swansea University, Department of Geography, Singleton Park, Swansea SA2 8PP, UK;5. Forest Research, Northern Research Station, Roslin, Midlothian EH26 9SY, UK;6. Sukachev Institute of Forest, Siberian Branch, Russian Academy of Sciences, Akademgorodok, Krasnoyarsk, 660036 Russia;1. State Key Laboratory of Vegetation and Environmental Change, Institute of Botany, Chinese Academy of Sciences, Beijing 100093, China;2. University of Chinese Academy of Sciences, Beijing 100049, China;3. College of Resources and Environmental Sciences, Xinjiang University, Urumqi 830002, China;4. Xinjiang Lidar Applied Engineering Technology Research Center, Urumqi 830002, China;5. Department of Geography, Faculty of Arts and Social Sciences, National University of Singapore, Singapore 117570, Singapore;6. Institute of Ecology, College of Urban and Environmental Science, Peking University, Beijing 100871, China;1. LiDAR Applications for the Study of Ecosystems with Remote Sensing (LASERS) Laboratory, Department of Ecosystem Science and Management, Texas A &M University, College Station, TX 77450, USA;2. Biospheric Sciences Lab, Code 618, NASA-GSFC, Greenbelt, MD 20771, USA;3. Geospatial Laser Applications and Measurements, Applied Research Laboratories, University of Texas at Austin, 10000 Burnet Rd, Austin, TX 78758, USA;4. NASA Goddard Space Flight Center, Greenbelt, MD 20771, USA;1. Department of Spatial Sciences, Faculty of Environmental Sciences, Czech University of Life Sciences Prague, Kamýcká 129, Praha-Suchdol, 165 00, Czech Republic;2. Institute for Environmental Studies, Faculty of Science, Charles University, Benátská 2, 12801 Prague 2, Czech Republic;3. Institute of Botany of the Czech Academy of Sciences, Zámek 1, CZ-252 43 Průhonice, Czech Republic;4. BIOME Lab, Department of Biological, Geological and Environmental Sciences, Alma Mater Studiorum University of Bologna, Bologna, Italy |
| |
Abstract: | 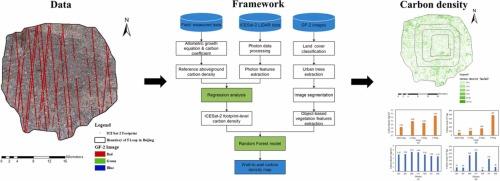 Accurately mapping carbon stocks of urban trees is necessary for urban managers to design strategies to mitigate climate change. However, the aboveground carbon stocks of urban trees are usually underestimated by passive remote sensing data because of the signal saturation problem. The research is the first attempt to develop a framework to map aboveground carbon density of trees in urban areas by synergizing Ice, Cloud and Land Elevation Satellite-2 (ICESat-2) LiDAR data with Gaofen-2 (GF-2) imagery. The framework consists of three key steps. First, we used a support vector machine classifier to classify GF-2 images and extracted urban tree regions. Second, we estimated the tree carbon density of ICESat-2 strips by developing a ICESat-2 photon feature-based aboveground carbon density estimation model. Third, we mapped the carbon density of urban trees by developing a synergistic model between ICESat-2 and GF-2 data based on an object-oriented method. We tested the approach for the areas within the fifth ring road of Beijing, China. The results showed that the 50th percentile height (PH50) of nighttime photons was a good predictor for estimating carbon density of urban trees, with a R2 of 0.69 and a Root Mean Square Error (RMSE) of 2.81 kg C m−2. Using the spectral features generated by GF-2 imagery, we could further extrapolate the carbon density estimated by ICESat-2 strip data to a full coverage of accurate mapping carbon density by urban trees, resulting in a R2 of 0.64 and a RMSE of 2.32 kg C m−2. The carbon stocks within the fifth ring road of Beijing were 8.28 × 108 kg in total, with the mean carbon density of 3.52 kg C m−2. Such estimations were larger than that of previous study using passive remote sensing data only, suggesting the integration of spaceborne LiDAR and spectral data could greatly reduce the underestimation of carbon stocks of urban trees. Our approach can more accurately estimate carbon stocks of urban trees and has the potential to be applicable in other cities. |
| |
Keywords: | Carbon stock Carbon density Urban tree Spaceborne LiDAR High-resolution imagery Remote sensing |
本文献已被 ScienceDirect 等数据库收录! |
|